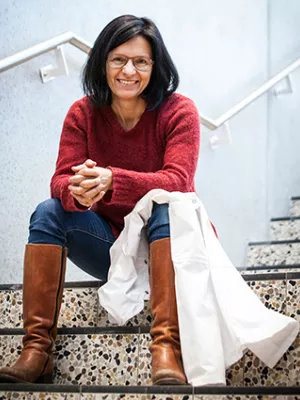
Emma Hammarlund
Research team manager
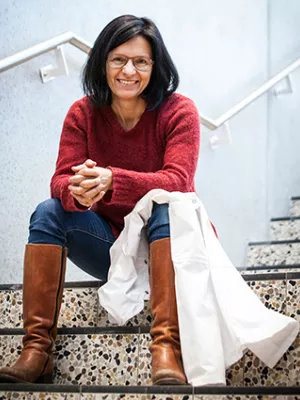
AlphaML : A clear, legible, explainable, transparent, and elucidative binary classification platform for tabular data
Author
Summary, in English
Leveraging the potential of machine learning and recognizing the broad applications of binary classification, it becomes essential to develop platforms that are not only powerful but also transparent, interpretable, and user friendly. We introduce alphaML, a user-friendly platform that provides clear, legible, explainable, transparent, and elucidative (CLETE) binary classification models with comprehensive customization options. AlphaML offers feature selection, hyperparameter search, sampling, and normalization methods, along with 15 machine learning algorithms with global and local interpretation. We have integrated a custom metric for hyperparameter search that considers both training and validation scores, safeguarding against under- or overfitting. Additionally, we employ the NegLog2RMSL scoring method, which uses both training and test scores for a thorough model evaluation. The platform has been tested using datasets from multiple domains and offers a graphical interface, removing the need for programming expertise. Consequently, alphaML exhibits versatility, demonstrating promising applicability across a broad spectrum of tabular data configurations.
Department/s
- StemTherapy: National Initiative on Stem Cells for Regenerative Therapy
- LUCC: Lund University Cancer Centre
- Division of Translational Cancer Research
- Molecular Cancer Research
- EpiHealth: Epidemiology for Health
- Molecular Evolution
- Stem Cell Center
Publishing year
2024-01
Language
English
Publication/Series
Patterns
Volume
5
Issue
1
Document type
Journal article
Publisher
Cell Press
Topic
- Bioinformatics (Computational Biology)
Keywords
- deep tabular learning
- drug sensitivity prediction
- DSML 3: Development/Pre-production: Data science output has been rolled out/validated across multiple domains/problems
- ensemble learning
- explainable AI
- feature selection
- hyperparameter optimization
- machine learning
- precision medicine
- TabNet
- XGBoost
Status
Published
Research group
- Molecular Cancer Research
- Molecular Evolution
ISBN/ISSN/Other
- ISSN: 2666-3899